rhinonet
The study explores the use of deep learning algorithms to develop a mobile application capable of classifying rhinoplasty procedures.
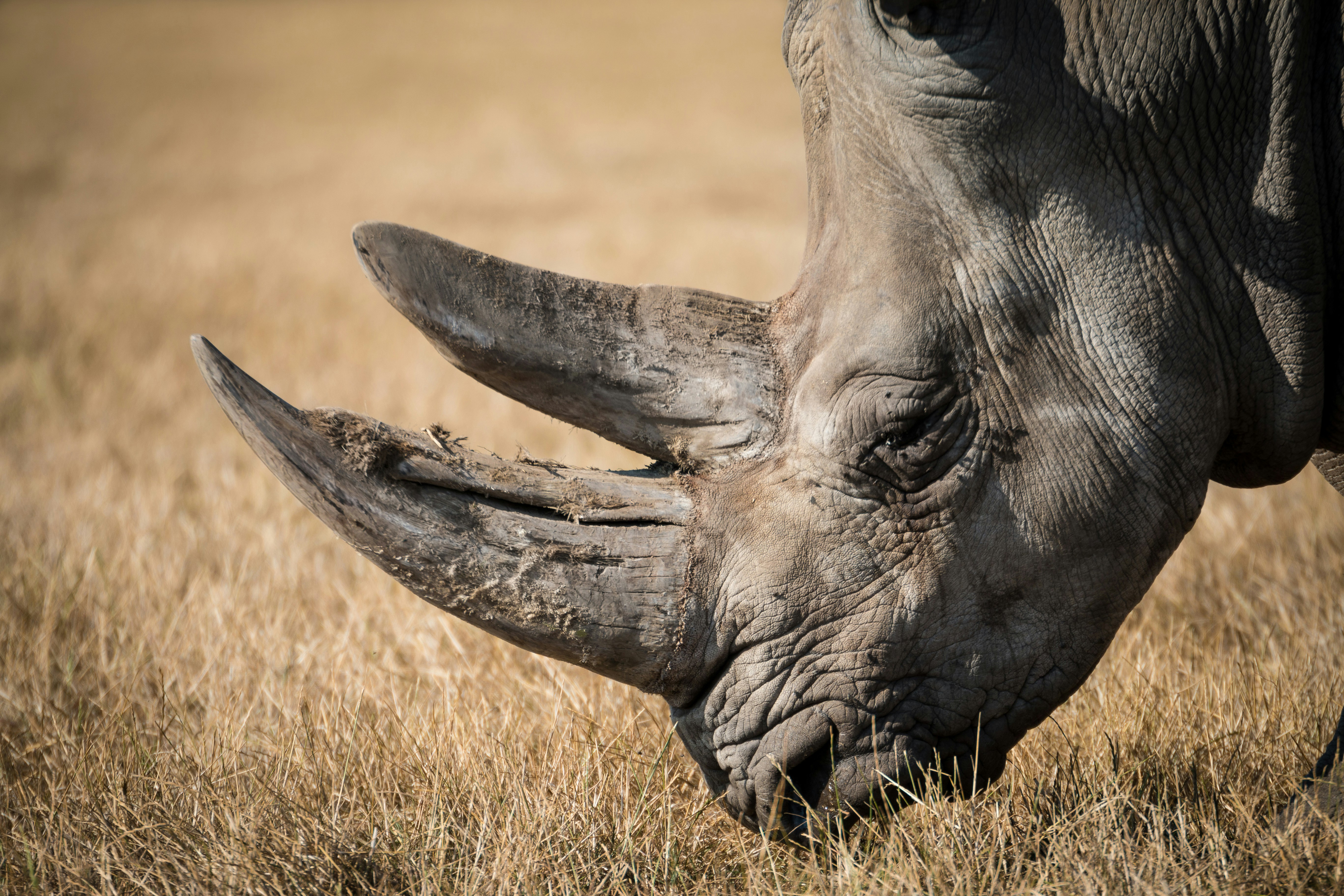
About the project
The study explores how deep learning can be used in plastic surgery to assist clinical decision-making. We developed a convolutional neural network (CNN) model to classify pre- and postoperative rhinoplasty images. The goal was to create a mobile app that could help surgeons evaluate surgical outcomes more efficiently by leveraging artificial intelligence (AI). The project highlights the growing integration of AI in medical fields, particularly in aesthetic and reconstructive surgery, to enhance diagnostic accuracy and streamline clinical workflows.
Key Features:
- Develop a mobile app powered by deep learning to classify rhinoplasty images scraped from RealSelf, a popular website where surgeons and patients share images.
- Used a CNN pre-trained on ImageNet to analyze pre- and postoperative photos.
- Aimed at assisting plastic surgeons and lay people in evaluating surgical results.

Results
The deep learning model could accurately distinguish between preoperative and postoperative rhinoplasty images. It correctly predicted rhinoplasty status in 85% of the test-set images. Sensitivity and specificity of model predictions were 0.840 (0.79-0.89) and 0.826 (0.77-0.88), respectively; the corresponding values for expert consensus predictions were 0.814 (0.76-0.87) and 0.867 (0.82-0.91). The model and humans performed with effectively equivalent accuracy in this classification task.
- The model achieved high classification accuracy in distinguishing rhinoplasty results.
- The model demonstrated promise for use in real-world clinical settings.
- There is potential expansion into other aesthetic procedures and clinical tasks (breast augmentation, botox).
